Rapidly changing algorithms and network architectures in the field of machine learning make Field Programmable Gate Array (FPGA) based designs an attractive solution for accelerating neural networks. However, the generic building blocks available in current FPGAs limit the acceleration that can be achieved. This technology, developed by researchers at The University of Texas at Austin, adds hard multiplier blocks into a field programmable gate array and provides for direct interconnect between them to create efficient larger matrix multipliers. This solution enables the design of faster and more space-efficient hardware accelerators for artificial neural network applications.
The hard matrix multiplier blocks can be laid out in various schemes in an FPGA chip (as shown above), depending on the market segment an FPGA chip is targeted towards. With this technology, an FPGA chip can perform a much larger number of mathematical operations per second per mm2 on the chip. A faster clock frequency, reduced area, and more power efficiency at performing matrix multiplication makes these modified field programmable gate arrays much more competitive for machine learning applications, compared to other options available such as Microsoft BrainWave, Google TPUs, or NVIDIA GPUs.
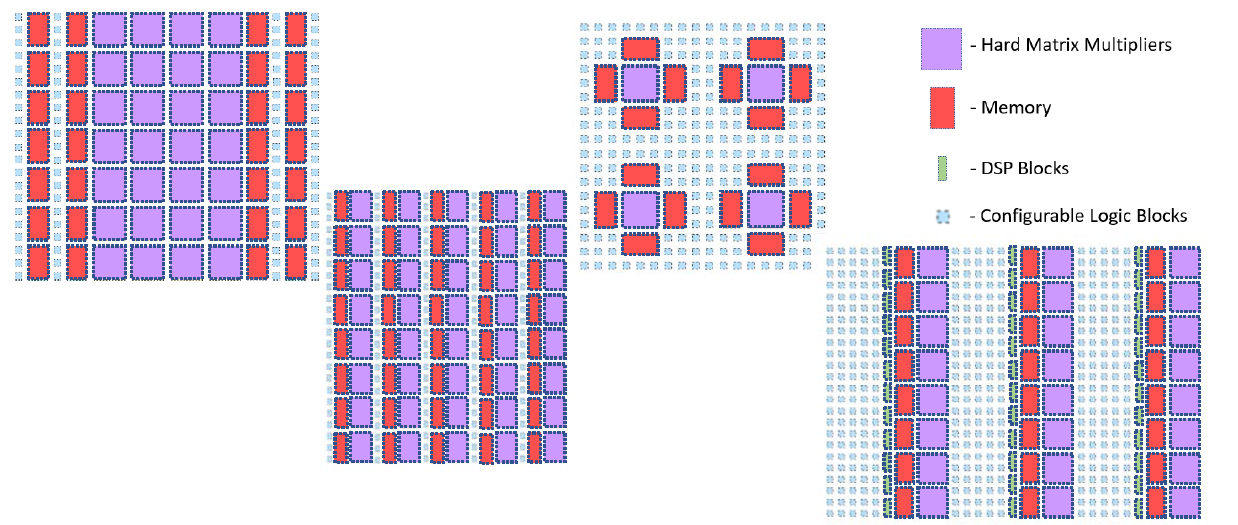
IP status: PCT application PCT/US2020/053209