Problem statement
Accurate 3D mapping of faults from seismic images is essential for seismic structural interpretation, reservoir characterization and well placement. Current methods for fault delineation depend on calculating attributes that estimate seismic reflection continuities and discontinuities. These methods are slow and can take days to process. The map obtained using these conventional methods does not provide desirable levels of detail.
Technology description
Researchers at The University of Texas at Austin have come up with an end-to-end Convolution Neural Network (CNN) approach to solve this problem. The neural network has been simplified to save GPU memory and computational time (for training and prediction) but still preserve the high performance for fault detection. The neural networks are trained using only 200 pairs of 3D synthetic seismic and fault volumes that are automatically generated by adding randomly adding folding, faulting, and noise in the volumes. Although trained by using only synthetic data sets, the neural network can accurately detect faults from 3D field seismic volumes that are acquired at totally different surveys. The method takes minutes compared to days needed by conventional methods. The approach provides a high accuracy and fast alternative to conventional methods currently in use (Fig. 1).
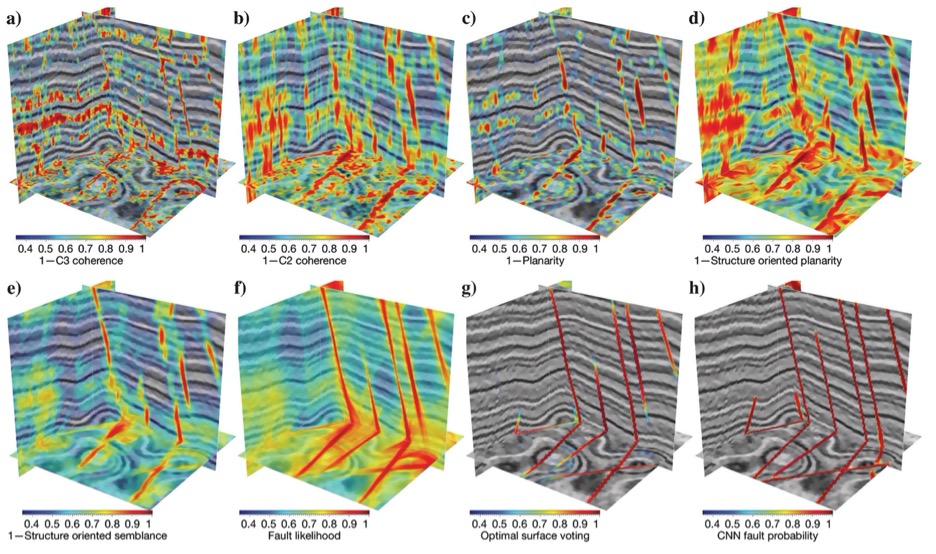
Fig 1. Fault detection on the synthetic validation volume by using seven commonly used methods (a-g) and (h) our CNN method. Compared with the methods of (a) C3 (Gersztenkorn and Marfurt, 1999), (b) C2 (Marfurt et al., 1999), (c) planarity (Hale, 2009), (d) structure-oriented planarity (Wu, 2017), (e) structure-oriented semblance (Hale, 2009), (f) the fault likelihood (Hale, 2013; Wu and Hale, 2016), and (g) optimal surface voting (Wu and Fomel, 2018) methods, our CNN method (h) achieves the best performance in obtaining an accurate, clean, and complete fault detection.
Benefits
- Fast: takes minutes compared to days for conventional methods
- Improved accuracy
- Versatile: the automatically generated synthetic training sets can be used for different formations.
Stage of development
This technology has been tested with field seismic images, and benchmarked against the state-of-the-art thinned fault likelihood attribute. These field data include Netherlands off-shore F3 block seismic data, the Clyde Petroleum Plc seismic images, date from the Costa Rica margin, northwest of the Osa Peninsula and the Campos Basin, offshore Brazil. Results from these comparisons show that the method works well and is very efficient, taking less than 3min to perform as compared to 1.5 hours with the conventional likelihood method. We are looking for partners to commercialize this product.
IP Position: US Patent Application
Publication: https://doi.org/10.1190/geo2018-0646.1, Wu et al. 2019